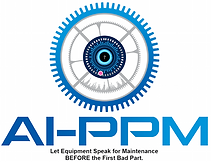
AI-PPM
Let Your Equipment Speak
AI-PPM Overview
Most plants use monitoring of the output (human or computer) through visual inspection for quality control - bad quality is the trigger for emergency maintenance, not planned maintenance. This generates waste and re-work costs, creates risks of charge-backs or other liability costs. The risk of damaging the credibility of the facility or company is potentially the most costly potential outcome of failure-based maintenance.
In most manufacturing facilities the machine operators are tasked to babysit quality. Unfortunately,, the operating of a machine, and meeting production goals affects their ability to be a good babysitter, and they often only look at the “kids” every hour or more.
AI-PPM is a great babysitter that looks at the kids every single minute, and communicates immediately any issues based on the rules provided by the parents by text.
The costs of a bad or distracted babysitter can be costly or tragic – only trust your company to the best of babysitters - AI-PPM.
The struggle for most US-based manufacturers is to find and retain quality employees. All the money, and time spent recruiting and training employees is for the purpose of developing in them expertise.
Their expertise develops over time for an operator to see, hear, feel, and smell changes in their machine to know something is wrong. When they leave the company, that experience and knowledge leaves too.
By using their minds to develop and refine the rules-based AI-PPM system that knowledge is retained forever. Avoid the expensive, but not quantified cost, of lost training and expertise.
The optimum maintenance program for any production machine would be to predict exactly the last quality part that could be produced on a machine, then shut down, and repair the machine. The process to achieve that is to use the collective knowledge of the operators in the facility to identify the root cause parameters that affect quality (in most industrial processes it will be 4 to 6 items that impact quality).
Statistical process control becomes insufficient for predictive preventative maintenance as it will monitor individual parameters, and quality failures will regularly be caused by the combination of multiple parameters degrading, but STILL BEING WITHIN ACCEPTED RANGES OF EACH PARAMETER.
AI Predictive Preventative Maintenance is a rules-based, continuous monitoring system for the degradation of mechanical systems so that maintenance, and operations are provided caution reports in advance of a machine failure. The system includes machine learning PLUS the collected experience of all operators that have worked in the facility over time to constantly refine decision rules.
A dataset of equipment parameters immediately prior to the production of bad parts is compared to a good dataset, and decision rules are established with the help of the operators, maintenance personnel. Once established, AI can be used to further refine the decision rules.
The optimum maintenance program for a facility would be to view each machine as a part in a larger machine, and to use rules-based decisions to plan facility maintenance – possible with AI-PPM.